Search Constraints
1 entry found
Number of results to display per page
Search Results
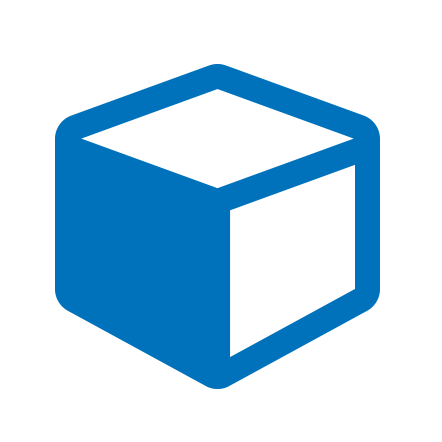
- Description:
- Weather-related research often requires synthesizing vast amounts of data that need archival solutions that are both economical and viable during and past the lifetime of the project. Public cloud computing services (e.g., from Amazon, Microsoft, or Google) or private clouds managed by research institutions are providing object data storage systems appropriate for long-term archives of such large geophysical data sets. , Current Status: Our research group no longer needs to maintain archives of High Resolution Rapid Refresh (HRRR) model output at the University of Utah since complete publicly-accessible archives of HRRR model output are now available from the Google Cloud Platform and Amazon Web Services (AWS) as part of the NOAA Open Data Program. Google and AWS store the HRRR model output in GRIB2 format, a file type that efficiently stores hundreds of two-dimensional variable fields for a single valid time. Despite the highly compressible nature of GRIB2 files, they are often on the order of several hundred MB each, making high-volume input/output applications challenging due to the memory and compute resources needed to parse these files. With support from the Amazon Sustainability Data Initiative, our group is now creating and maintaining HRRR model output in an optimized format, Zarr, in a publicly-accessible S3 bucket- hrrrzarr. HRRR-Zarr contains sets for each model run of analysis and forecast files sectioned into 96 small chunks for every variable. The structure of the HRRR-Zarr files are designed to allow users the flexibility to access only the data they need through selecting subdomains and parameters of interest without the overhead that comes from accessing numerous GRIB2 files. , and History: This effort began in 2015 to illustrate the use of a private cloud object store developed by the Center for High Performance Computing (CHPC) at the University of Utah. We began archiving thousands of two-dimensional gridded fields (each one containing over 1.9 million values over the contiguous United States) from the High-Resolution Rapid Refresh (HRRR) data assimilation and forecast modeling system. The archive has been used for retrospective analyses of meteorological conditions during high-impact weather events, assessing the accuracy of the HRRR forecasts, and providing initial and boundary conditions for research simulations. The archive has been accessible interactively and through automated download procedures for researchers at other institutions that can be tailored by the user to extract individual two-dimensional grids from within the highly compressed files. Over a thousand users have voluntarily registered to use the HRRR archive at the University of Utah. Our archive has grown to over 130 Tbytes of model output but we no longer need to continue that effort since the GRIB2 files are available now via Google and AWS. As mentioned above, we now provide much of the same information in an alternative format that is appropriate particularly for machine-learning applications.
- Keyword:
- data assimilation, Zarr, weather, forecasts, high resolution rapid refresh, and numerical weather prediction
- Subject:
- atmospheric science
- Creator:
- Horel, John and Blaylock, Brian
- Contributor:
- University of Utah Center for High Performance Computing, NOAA Earth Systems Research Laboratory, Amazon Open Data Program, and NOAA Environmental Modeling Center
- Depositor:
- BRIAN MCBRIDE
- Owner:
- JOHN HOREL
- Based Near Label Tesim:
- Alaska, Alaska, United States and United States, , United States
- Language:
- binary and English
- Date Uploaded:
- 07/10/2019
- Date Modified:
- 04/18/2024
- Date Created:
- 2015-04-18 to 2019-07-10
- License:
- CC BY – Allows others to use and share your data, even commercially, with attribution.
- Resource Type:
- Dataset
- Identifier:
- https://dx.doi.org/10.7278/S5JQ0Z5B