Search Constraints
Filtering by:
Location
Salt Lake City, Utah, United States
Remove constraint Location: Salt Lake City, Utah, United States
« Previous |
1 - 10 of 34
|
Next »
Number of results to display per page
Search Results
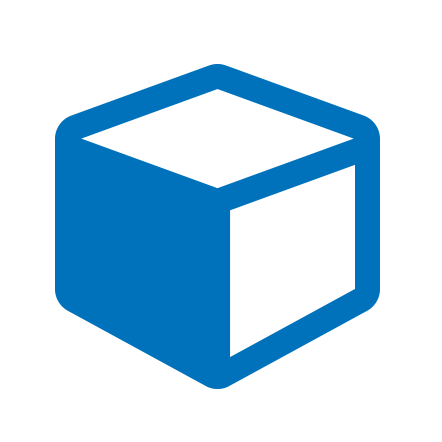
- Description:
- This is a data set for generating current densities used for the validation of two methods. Similarly, it gives the electric fields for the 80-minute validation of the two methods. Furthermore, the partial transfer function method calculated electric fields are also deposited in this dataset. Similarly, the spectrum of each source and impulse response obtained from the FDTD model are also included. Finally, the electric fields were obtained for 8 hours using the PTF method.
- Keyword:
- FDTD, Geoelectric fields, Partial transfer function, and Long-time span
- Subject:
- geoelectricity and finite difference time domain method
- Creator:
- Sharma Paneru, Prashanna
- Owner:
- Based Near Label Tesim:
- Salt Lake City, Utah, United States
- Language:
- English
- Date Uploaded:
- 11/08/2024
- Date Modified:
- 11/11/2024
- Date Created:
- 2024-01-01 to 2024-11-08
- License:
- Public Domain – This data is free of copyright restrictions (e.g. government sponsored data).
- Resource Type:
- Dataset
- Identifier:
- https://doi.org/10.7278/S5d-ev5n-d1v5
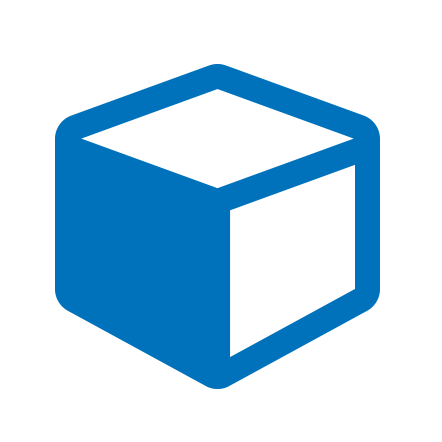
- Description:
- Whole-cell recordings from the anuran inferior colliculus. The data is averaged & representative whole-cell recordings, stats and code used for the analysis.
- Keyword:
- counting neurons, estimating elapsed time, and decoding information
- Subject:
- Counting, time perception, whole-cell recordings, and interval counting neurons
- Creator:
- Alluri, Rishi K. and Rose, Gary J.
- Contributor:
- Vasquez-Opazo, Gustavo A., Odom, Stephen E., Graham, Jalina A., Leary, Christopher J., Hanson, Jessica L. , Wilkerson, Jeremy, Palaparthi, Anil, Luong, Kyphuong, McDowell, Jamie, and Mukhopadhyay, Anwesha
- Owner:
- Based Near Label Tesim:
- Salt Lake City, Utah, United States
- Language:
- English
- Date Uploaded:
- 07/25/2024
- Date Modified:
- 08/08/2024
- Date Created:
- 2005-01-07 to 2022-12-07
- License:
- CC BY NC - Allows others to use and share your data non-commercially and with attribution.
- Resource Type:
- Dataset
- Identifier:
- http://doi.org/10.7278/S5d-0ndd-9r1n
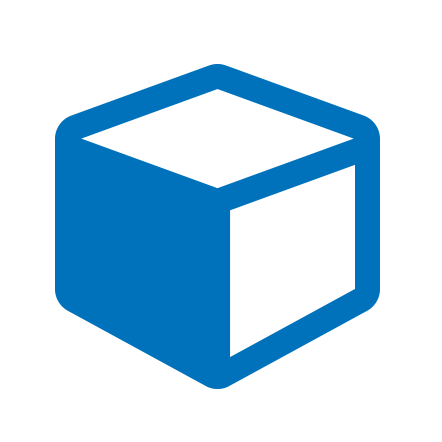
- Description:
- Data were collected from the free online available International Ionosphere Reference (IRI) database ( https://kauai.ccmc.gsfc.nasa.gov/instantrun/iri) for January 1, 2020. The data were then interpolated from 1km to our desired resolution of 0.1km. This is is profile used to simulate the 3D FDTD models to observe the propagation of power line harmonic radiation through the ionosphere.
- Keyword:
- Ionosphere and Inhomogeneous Ionosphere
- Subject:
- FDTD (mathematics), plasmas (physics) , and ionospheric electron density
- Creator:
- Pedgaonkar, Apoorva
- Owner:
- Based Near Label Tesim:
- Salt Lake City, Utah, United States
- Language:
- English
- Date Uploaded:
- 07/12/2024
- Date Modified:
- 09/06/2024
- Date Created:
- 2020-01-20
- License:
- Public Domain – This data is free of copyright restrictions (e.g. government sponsored data).
- Resource Type:
- Dataset
- Identifier:
- http://doi.org/10.7278/S5d-hpp0-g1wy
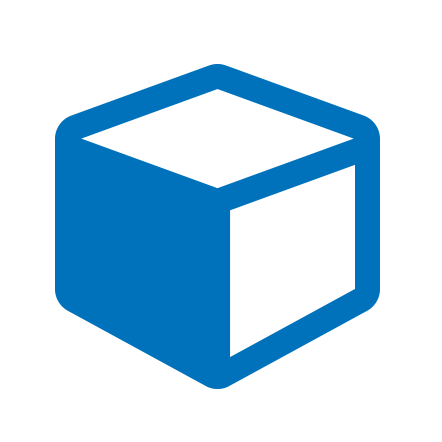
- Description:
- The dataset contains velocity measurements along the fiber optic cable connecting the University of Utah campus to the University of Utah Downtown data center (875 West Temple, Salt Lake City, UT). The data has been collected using the Distributed acoustic sensing (DAS) system that records the vibration signals along 8.4 km long optical fiber every 4.9-m interval with a sampling rate of approximately 1000 Hz. The fiber is mainly installed along the red line of TRAX, which is the light rail system of the Utah Transit Authority. The route intersects the East Bench fault, which is known as an active fault segment of the Wasatch Fault zone. Although no earthquake signals were detected, the velocity data converted to strain rate clearly show the operation of trains between the stations at 450 S Main Street and 900 South 200 West. Analysis of this dataset is expected to provide insights into seismic velocities at shallow depths and structures associated with fault scarps. and See README file for data retrieval instructions.
- Keyword:
- seismology, urban seismology, distributed acoustic sensing, fiber optic cable, and strain rate
- Subject:
- Geophysics, Seismology, Seismology--Observations, and Surface fault ruptures
- Creator:
- Kim, HyeJeong and Lin, Fan-Chi
- Contributor:
- Chambers, Derrick
- Owner:
- Kaylee Alexander
- Based Near Label Tesim:
- Salt Lake City, Utah, United States
- Language:
- English
- Date Uploaded:
- 07/01/2024
- Date Modified:
- 11/05/2024
- Date Created:
- 2023-05-24 to 2023-05-26 (period 1), 2023-08-03 to 2023-08-11 (period 3), 2023-12-22 to 2024-01-02 (period 4), and 2023-06-29 to 2023-07-10 (period 2)
- License:
- CC BY NC - Allows others to use and share your data non-commercially and with attribution.
- Identifier:
- https://doi.org/10.7278/S5d-kgxx-ev8y
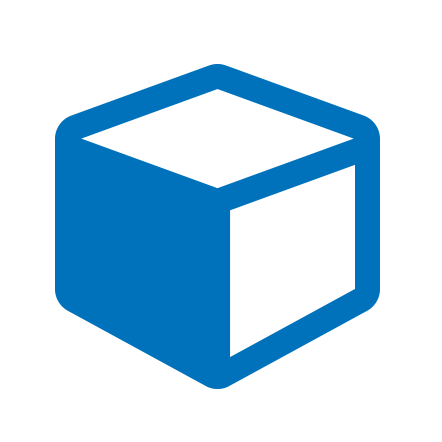
5. Pacific Low Cloud Occurrence Variability Associated with ENSO as Observed by CloudSat and CALIPSO
- Description:
- As strong cooling agents in the climate system, marine low-level clouds are an important component of the climate system. Demonstrating how marine low-level clouds respond to anomalies in the atmospheric general circulation in the present climate has the potential to be illustrative of how low clouds might change in a future climate. We examine how thermodynamic factors that control low cloud occurrence change during an ENSO cycle and then how low clouds observed by the CloudSat and CALIPSO satellites vary. In addition to the well-known decrease in marine low clouds in the Northeast Pacific during El Niño onset in June, July and August (JJA), we also find significant increases in the low cloud occurrence on the flanks of the anomalously warm water in the Equatorial Central Pacific during December, January and February (DJF). These low cloud changes are linked to measurable changes in the Earth’s energy budget with net warming of the Earth system during JJA and cooling of the Earth system during DJF. This is the python code to create the figures for the paper about the above research.
- Keyword:
- CloudSat , CALIPSO, El Niño–Southern Oscillation (ENSO), and marine
- Subject:
- ENSO
- Creator:
- Gombert, Peter M., Strong, Courtenay, and Mace, Gerald G. (Jay)
- Owner:
- Sally Benson
- Based Near Label Tesim:
- Salt Lake City, Utah, United States
- Language:
- Python and English
- Date Uploaded:
- 06/18/2024
- Date Modified:
- 09/09/2024
- Date Created:
- 2023-06-01 to 2024-06-01 (collected) and 2007-2018 (created)
- License:
- CC BY NC - Allows others to use and share your data non-commercially and with attribution.
- Resource Type:
- Software or Program Code
- Identifier:
- https://doi.org/10.7278/S5d-64j3-1n2n
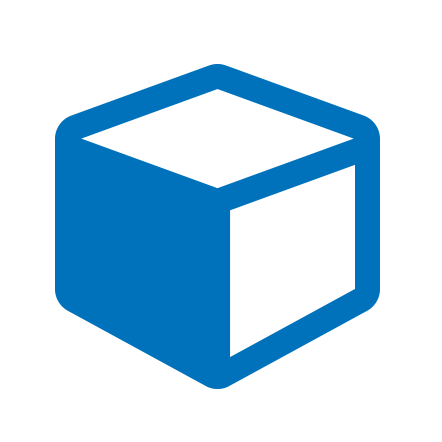
6. Intraoperative Characterization of Cardiac Tissue: The Potential of Light Scattering Spectroscopy
- Description:
- The spectral data required to reproduce the results from the paper "Intraoperative characterization of cardiac tissue: the potential of light scattering spectroscopy," published in the Journal of Biomedical Optics.
- Keyword:
- Machine Learning, Light Scattering Spectroscopy, and Cardiac Tissue Characterization
- Subject:
- Spectrum Analysis, Machine Learning, and Congenital Abnormality
- Creator:
- Cottle, Brian, Sachse, Frank, and Hitchcock, Robert
- Contributor:
- Tiwari, Sarthak
- Owner:
- Brian Cottle
- Based Near Label Tesim:
- Salt Lake City, Utah, United States
- Language:
- English
- Date Uploaded:
- 05/29/2024
- Date Modified:
- 07/01/2024
- Date Created:
- 2021-04-01 to 2023-08-31
- License:
- CC BY NC - Allows others to use and share your data non-commercially and with attribution.
- Resource Type:
- Dataset
- Identifier:
- https://doi.org/10.7278/S5d-1mxa-ffa0
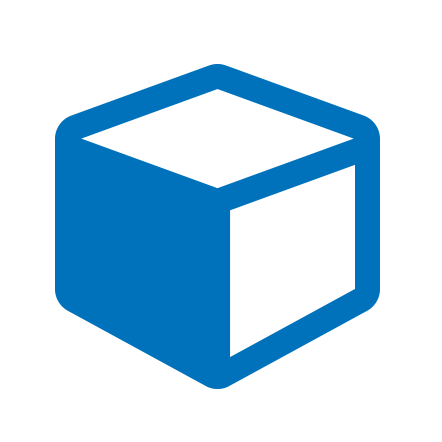
- Description:
- The microbiology data represents the microorganisms recovered during the study period at the University of Utah hospital from samples collected from patients, environmental surfaces, and healthcare personnel (HCP) hands using premoistened sponges. Patient samples were collected daily from the axilla, groin, and perianal areas or stool. Environmental samples were collected daily from room surfaces and unit common areas (such as bed rails, overbed tables, door handles, computer keyboards, and other high-touch areas). HCP hands were periodically sampled upon HCP exit from a patient room after engaging in health care activities. Samples were collected from the 20-bed University of Utah Hospital Cardiovascular ICU (CVICU) over a 54 day period. The information from these datasets can be used to understand how different organisms appear and move throughout a hospital ward over a period of time.
- Keyword:
- patient sampling, microbiology, multidrug-resistant organisms, healthcare worker hand sampling, and environmental sampling
- Subject:
- microbiology
- Creator:
- Rubin, Michael , Leecaster, Molly, and Haroldsen, Candace
- Contributor:
- Kristina Stratford and Tavis Huber
- Owner:
- Michael Rubin
- Based Near Label Tesim:
- Salt Lake City, Utah, United States
- Language:
- English
- Date Uploaded:
- 12/26/2023
- Date Modified:
- 01/04/2024
- Date Created:
- 2018-03-01 to 2018-04-28
- License:
- CC BY NC - Allows others to use and share your data non-commercially and with attribution.
- Resource Type:
- Dataset
- Identifier:
- http://doi.org/10.7278/S50d-n2s8-h8jk
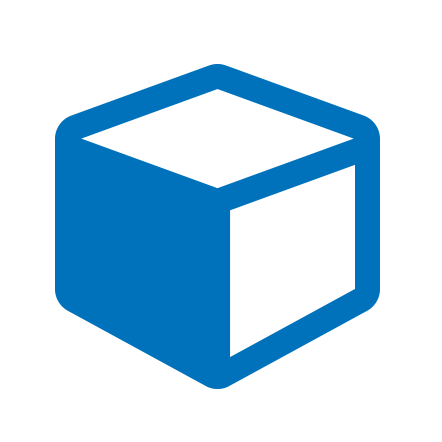
- Description:
- This dataset contains room occupancy during the study period at University of Utah hospital. Admission, Discharge, and Transfer (ADT) data is captured in participating hospitals to characterize room occupancy and non-occupancy in wards. These data are pulled from multiple sources collected during the study by study staff as well as harvested EHR data. Data were adjudicated and compiled into one comprehensive file. Data manipulation included redaction of dates, replaced with study days 1-n, as well as transformation from long format to wide for ease of use.
- Keyword:
- bed occupancy, transfer, discharge, ADT, and admission
- Subject:
- bed occupancy
- Creator:
- Haroldsen, Candace, Rubin, Michael, and Leecaster, Molly
- Contributor:
- Huber, Tavis and Stratford, Kristina
- Owner:
- Michael Rubin
- Based Near Label Tesim:
- Salt Lake City, Utah, United States
- Language:
- English
- Date Uploaded:
- 12/26/2023
- Date Modified:
- 11/05/2024
- Date Created:
- 2018-01-01 to 2018-12-31
- License:
- CC BY NC - Allows others to use and share your data non-commercially and with attribution.
- Resource Type:
- Dataset
- Identifier:
- www.doi.org/10.7278/S50d-6wz0-jk8j
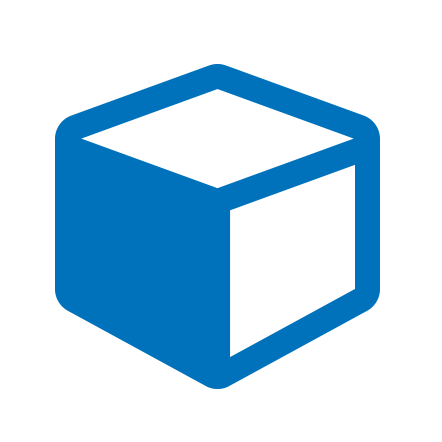
- Description:
- The objective of using the wireless sensors was to improve understanding of the heterogeneity of healthcare worker (HCW) contact with patients and the physical environment in patients’ rooms. The framework and design were based on contact networks with a) nodes defined by HCW’s, rooms, and items in the room and b) edges defined by HCW’s in the room, near the bed, and touching items. Nodes had characteristics of HCW role and room number. Edges had characteristics of day, start time, and duration. Thus, patterns and heterogeneity could be understood within contexts of time, space, roles, and patient characteristics. At the University of Utah Hospital Cardiovascular ICU (CVICU), a 20-bed unit, we collected data for 54 days. HCW contact with patients was measured using wireless sensors to capture time spent in patient rooms as well as time spent near the patient bed. HCW contact with the physical environment was measured using wireless sensors on the following items in patient rooms: door, sink, toilet, over-bed table, keyboard, vital signs monitor touchscreen, and cart. HCW’s clipped a sensor to their clothing or lanyard. This dataset contains cleaned event-level data processed from sensor pings of RFD reads between healthcare worker worn sensors and environmental sensors placed in facility using methods described in the "Data Cleaning Steps" section.
- Keyword:
- patient contact and wireless sensors
- Subject:
- cardiology
- Creator:
- Leecaster, Molly, Rubin, Michael, and Haroldsen, Candace
- Contributor:
- Huber, Tavis and Stratford, Kristina
- Owner:
- Michael Rubin
- Based Near Label Tesim:
- Salt Lake City, Utah, United States
- Language:
- English
- Date Uploaded:
- 12/26/2023
- Date Modified:
- 11/05/2024
- Date Created:
- 2018-01-01 to 2018-12-31
- License:
- CC BY NC - Allows others to use and share your data non-commercially and with attribution.
- Resource Type:
- Dataset
- Identifier:
- www.doi.org/10.7278/S50d-hmxz-4bf1
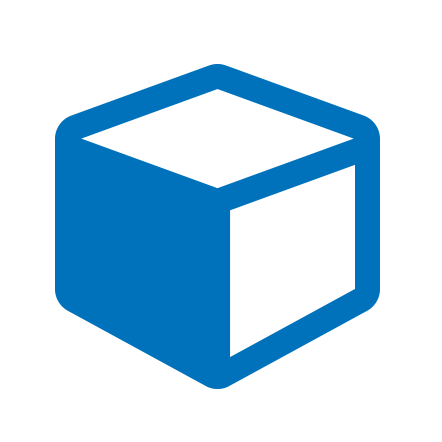
- Description:
- The objective of using the wireless sensors was to improve understanding of the heterogeneity of healthcare worker (HCW) contact with patients and the physical environment in patients’ rooms. The framework and design were based on contact networks with a) nodes defined by HCW’s, rooms, and items in the room and b) edges defined by HCW’s in the room, near the bed, and touching items. Nodes had characteristics of HCW role and room number. Edges had characteristics of day, start time, and duration. Thus, patterns and heterogeneity could be understood within contexts of time, space, roles, and patient characteristics. At the University of Utah Hospital Cardiovascular ICU (CVICU), a 20-bed unit, we collected data for 54 days. HCW contact with patients was measured using wireless sensors to capture time spent in patient rooms as well as time spent near the patient bed. HCW contact with the physical environment was measured using wireless sensors on the following items in patient rooms: door, sink, toilet, over-bed table, keyboard, vital signs monitor touchscreen, and cart. HCW’s clipped a sensor to their clothing or lanyard. This dataset contains cleaned sensor pings of RFD reads between healthcare worker worn sensors and environmental sensors placed in facility using methods described in the "Data Cleaning Steps" section.
- Keyword:
- patient contact and wireless sensors
- Subject:
- cardiology
- Creator:
- Rubin, Michael, Haroldsen, Candace, and Leecaster, Molly
- Contributor:
- Huber, Tavis and Stratford, Kristina
- Owner:
- Michael Rubin
- Based Near Label Tesim:
- Salt Lake City, Utah, United States
- Language:
- English
- Date Uploaded:
- 12/26/2023
- Date Modified:
- 11/05/2024
- Date Created:
- 2018-01-01 to 2018-12-31
- License:
- CC BY NC - Allows others to use and share your data non-commercially and with attribution.
- Resource Type:
- Dataset
- Identifier:
- www.doi.org/10.7278/S50d-twbh-955q
- « Previous
- Next »
- 1
- 2
- 3
- 4